Plan, Posture and Go:
Towards Open-World Text-to-Motion Generation
Exemplary motions generated by our proposed PRO-Motion system. Different from conventional models trained on paired text-motion data, our PRO-Motion can generate 3D human motion with global body translation and rotation from open-world text prompts, such as "Jump on one foot" and "Experiencing a profound sense of joy".
Abstract
Conventional text-to-motion generation methods are usually trained on limited text-motion pairs, making them hard to generalize to open-world scenarios. Some works use the CLIP model to align the motion space and the text space, aiming to enable motion generation from natural language motion descriptions. However, they are still constrained to generate limited and unrealistic in-place motions. To address these issues, we present a divide-and-conquer framework named PRO-Motion, which consists of three modules as motion planner, posture-diffuser and go-diffuser. The motion planner instructs Large Language Models (LLMs) to generate a sequence of scripts describing the key postures in the target motion. Differing from natural languages, the scripts can describe all possible postures following very simple text templates. This significantly reduces the complexity of posture-diffuser, which transforms a script to a posture, paving the way for open-world generation. Finally, go-diffuser, implemented as another diffusion model, estimates whole-body translations and rotations for all postures, resulting in realistic motions. Experimental results have shown the superiority of our method with other counterparts, and demonstrated its capability of generating diverse and realistic motions from complex open-world prompts such as
Method
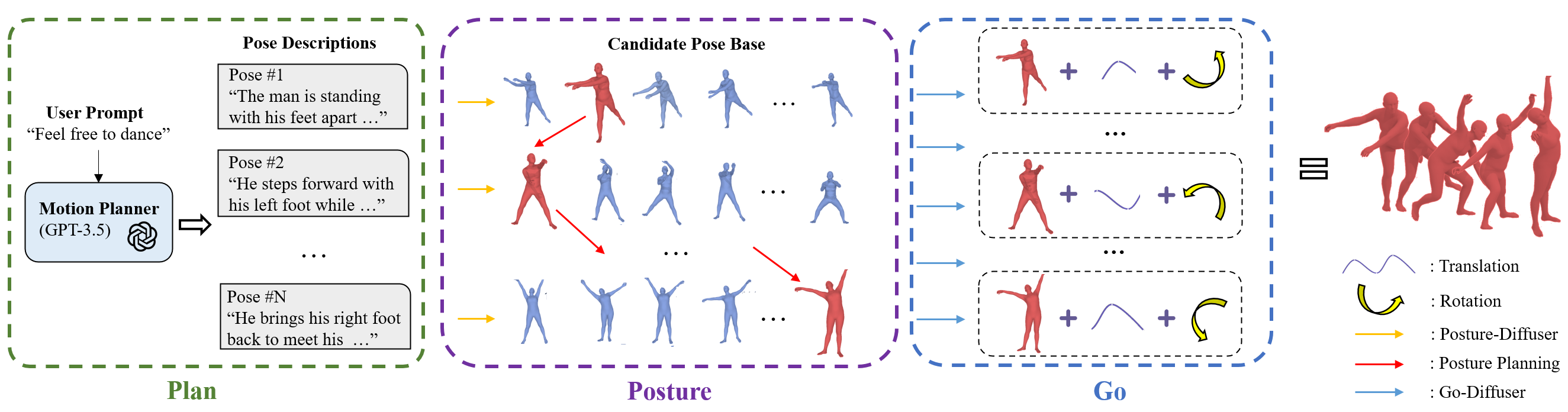
Illustration of our framework for open-world text-to-motion generation from language. Specifically, we employ the large language models as the motion planner to plan pose scripts. Then, our
Gallery
The guy is imitating a singing star.
Jump on one foot.
Experiencing a profound sense of joy.
Bury one's head and cry, and finally crouched down.
Comparisons
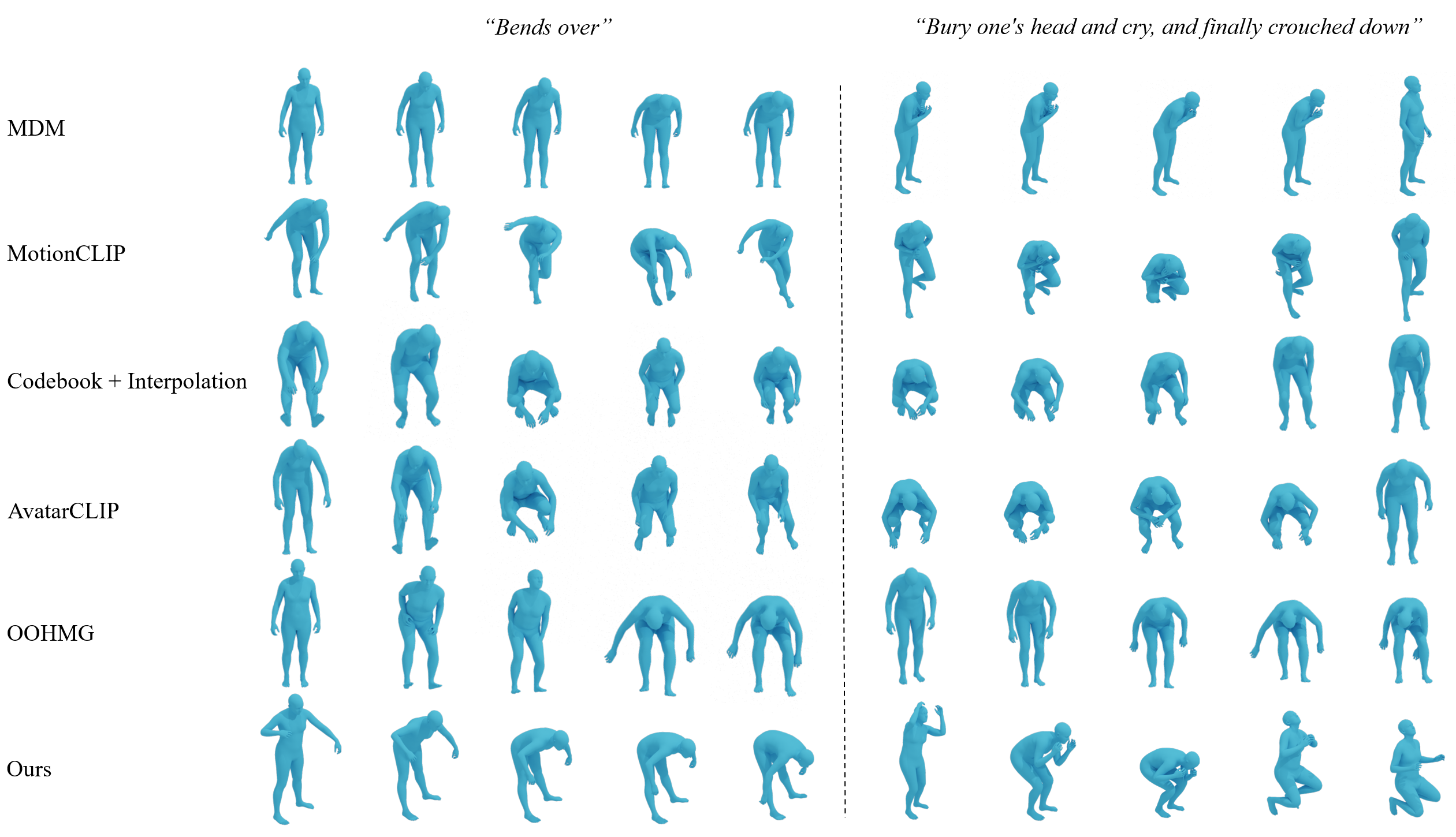
Comparison of our method with previous text-to-motion generation methods.
Posture-Diffuser
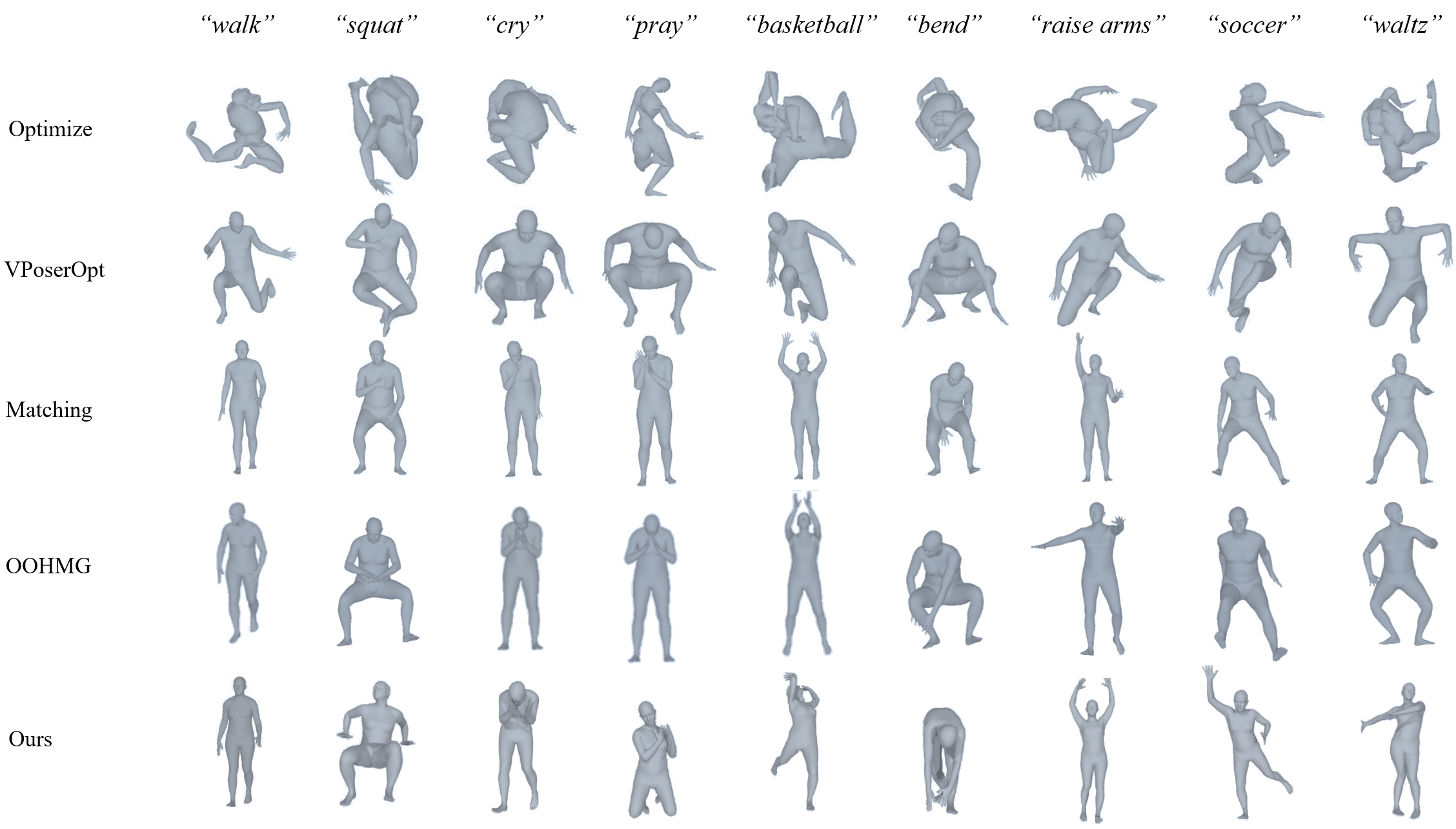
Comparison of our method with previous text-to-pose generation methods.
Go-Diffuser
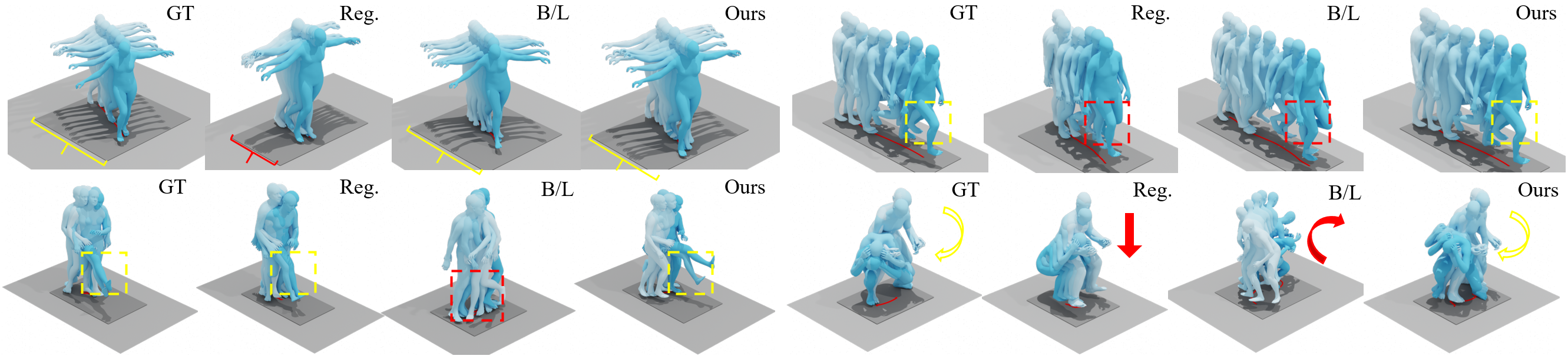
Comparison of our method with other pose-to-motion generation methods. Yellow color represents details that need attention, and red color represents inaccuracies.
New Advantages - Precise Pose Control
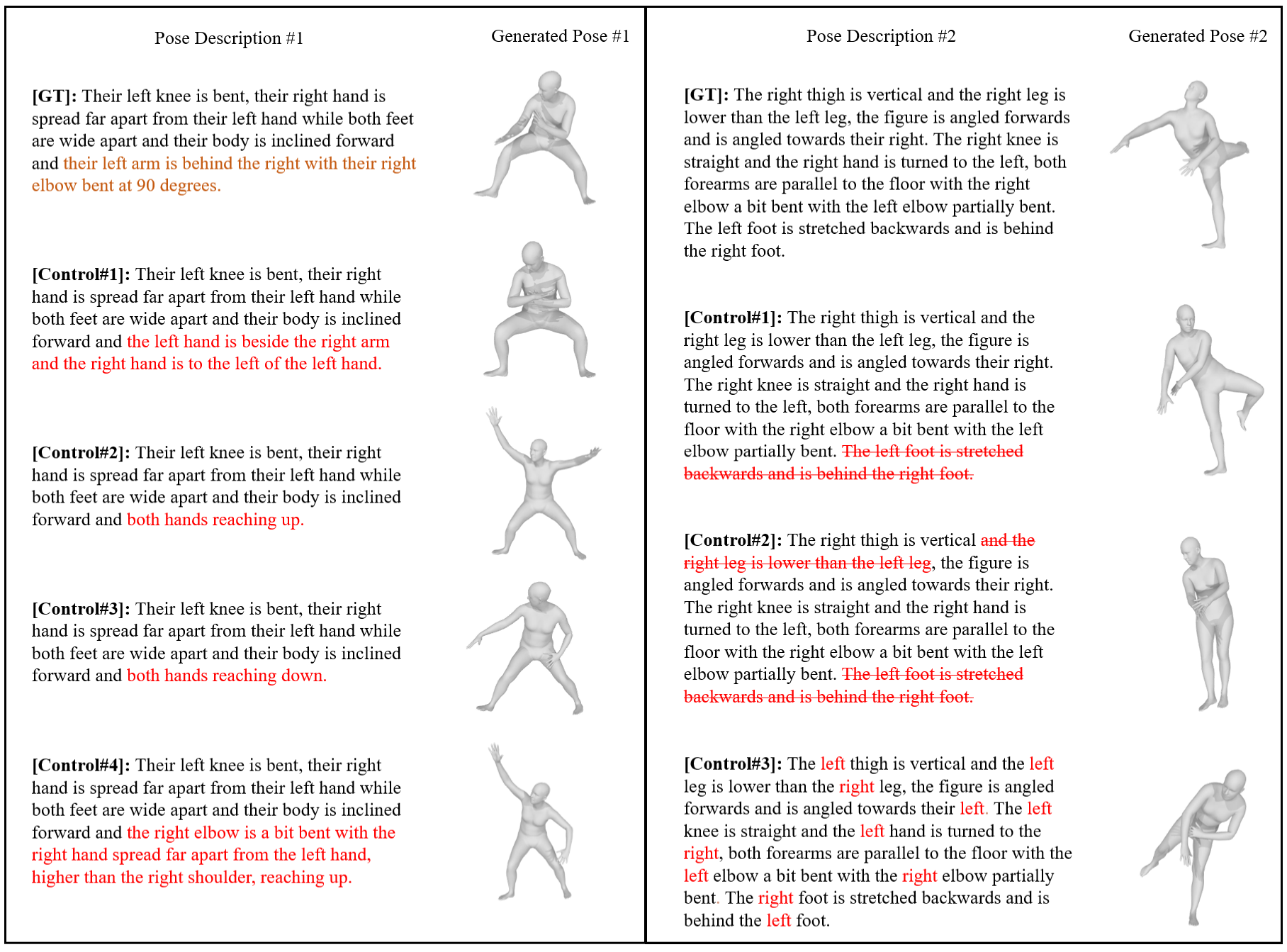
Examples of Precise Pose Control. In the first example #1, we control the position of hands, with the original and modified hand descriptions represented in brown and brown respectively. In the second example #2, "The" represents deleting the description. To determine whether the description of a body part is the reason for the proper positioning of that body part, we removed the corresponding descriptions to see if the body joints would change as a result of this operation. We test the model's understanding of the left and right sides of the body.
Acknowledgements
The website template was borrowed from Michaël Gharbi and Jon Barron.